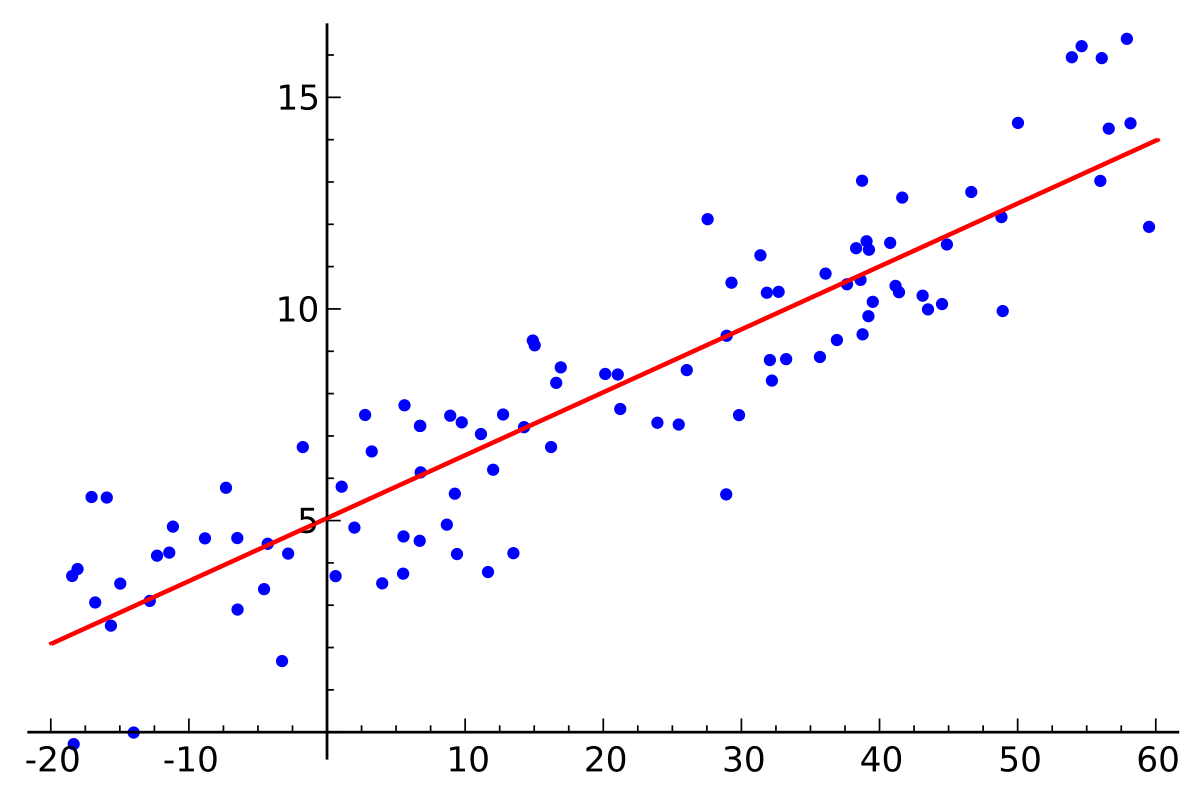
The main focus of the course is on the practical side of using regression to address common methodological, statistical and data issues that arise in the social sciences. The course begins with a brief review of the principles of multiple regression. It then examines the specific issues and problems that arise from its application, considers appropriate approaches to address the problems, and how to interpret the results that it produces.
These issues include:
• regression diagnostics to detect potential violations of multiple regression's assumptions
• how to identify the presence of multicollinearity and what to do about it
• establishing the existence of non-linear relationships and identifying the transformation procedures necessary to apply to the data
• how to treat missing data, particularly the use of multiple imputation (MI) approaches
• how to incorporate and interpret interactions and moderator variables
• how to model and test for mediating variables, including the classic Baron-Kenny approach, the Sobel test, and more modern approaches
• how to estimate model with binary and ordinal dependent variables (including binary logistic regression and ordered probit)
• how to estimate models with simultaneity (“two-way” causation) using two-stage least squares.
The course concludes by examining the relationship between multiple regression and structural equation modeling (SEM) with latent variables and compares the results of regression and SEM analyses.
This course may run in a computer lab, or you may be advised to bring your own laptop with preferred software.
We will let you know in advance.
If this course takes place in a computer lab, you are still welcome to bring your own laptop pre-loaded with whatever statistical software package you use (SPSS, SAS, Stata, or R) and, where appropriate, your own datasets. You will have an opportunity to analyse your own data and discuss the output.
Knowledge of elementary statistical techniques and some knowledge of the principles of multiple regression at a level comparable to that provided by the Fundamentals of Multiple Regression course, and basic knowledge and skills of a statistical package (SPSS, SAS, Stata or R).
An additional, optional resource which covers multiple regression and basic structural equation modeling is:
- Timothy Keith, Multiple Regression and Beyond (Allyn & Bacon, 2005),
David did an absolutely wonderful job of explaining regression (Summer 2020)
The course was very thorough and the course teacher very passionate. I found it useful and the notes really helpful. (Summer 2019)
The course content is very useful for developing my PHD research work analysis models and also gave me ideas for more new analysis on existing data sets. (Summer 2019)
Nice balance of activities. (Summer 2016)
Course notes provided were very well written and comprehensive. David gave us a good mix of theoretical and practical information. (Summer 2016)
It is just what I needed at this time, formulate models for my research, got the idea now – happy. (Summer 2014)
The instructor's bound, book length course notes will serve as the course texts.